“Current data-based methods of analysis and predictive models are insufficient – big data is able to remedy this. ”
– Dr. Alexander Lenk
Pass/fail decisions by faculty are mostly based on the ability of students to pass or fail prior to (Angoff) or post standard setting (Borderline Regression Analysis) criteria developed by faculty. Standard setting is a critical part of educational, licensing and certification testing. But outside of the cadre of practitioners, this aspect of test development is not well understood. Standard setting is the methodology used to define levels of achievement or proficiency and the cut-scores corresponding to those levels. A cut-score is simply the score that serves to classify the students whose score is below the cut-score into one level and the students whose score is at or above the cut-score into the next and higher level.
Prior standard setting in education is linked to the assumption of the ability/proficiency of students in performing a certain task according to specific level. However, whether a student or applicant is competent in performing a certain task/job depends on the combination of knowledge, skills and attitudes. Educational decision making is about the decision of whether a student/applicant is able to perform and likely to be proficient in their future job. Data analysis must be a part of the assessment process.
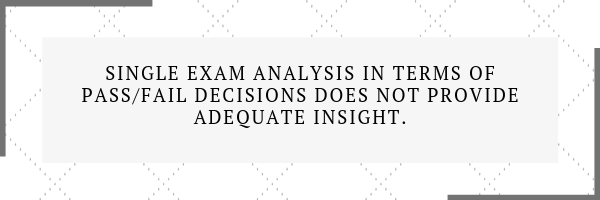
Are cut-scores appropriately set?
Unless cut-scores are appropriately set, the results of any assessment could come into question. Do single cut-scores of examinations predict future outcome in terms of whether a professional is fit for a job? Clearly, unless the cut-scores are appropriately set, the results of the assessment could come into question as they may be used to predict future performance such as, proficiency levels. For that reason, standard setting is a critical component of the test development process and, moreover, the interpretation and decisions around these cut-scores need to be well understood.
What is the observed score and the true score?
If we standard set an examination we assume the observed score represents the true ability of the examinee to pass the test. However, the observed score of the examinee represents the ability to do the test at that point in time. The observed score, the score we obtained from a student, applicant or examinee, represents a score out of the universe of potential scores. Furthermore, whether examinees pass or fail for a single item or a subset of items, tasks depend on the difficulty of these items, for example the mood of the examiner observing the examinee performing the task.
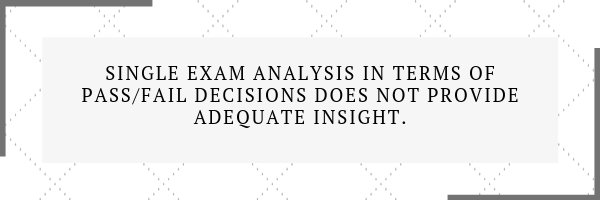
If we don’t take the error around the true score into account we might make the wrong decision on whether that examinee should pass or fail that specific test. Not to mention a combination of tests (and error around those tests) used to determine future performance or likely proficiency in certain high stakes jobs. By identifying the sources of error through smart data analysis we are able to improve our test battery/portfolio. Smart data analysis provides insight into whether an examinee is likely to be proficient in their future career.
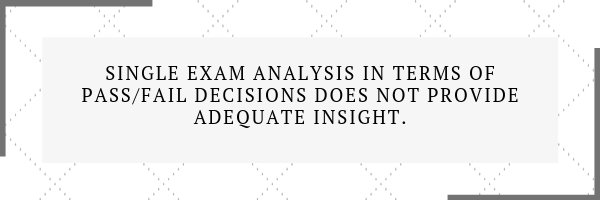
Are cut-scores appropriately interpreted?
The SEM represents the Quality Assurance of your data set. The observed score plus or minus the SEM provides insight in the confidentiality interval in which the true ability of the candidate to pass or fail the test can be expected. Using 1 SEM, you are 68% sure the true score will be in between the observed score plus or minus 1 SEM. In other words, the examinee’s true ability on the test is in between the observed score plus 1 SEM and the observed score minus 1 SEM. There is still a 32% chance false positive students have passed the test where they should not pass, coming through the test for the wrong reasons. The same goes for false negatives. In this case, students failed but their true ability score could have been a pass score if the test would have been repeated multiple times.
In terms of high stakes decisions in Medicine, Health Sciences, the Police and Army Forces and the Ambulance Service, it would be preferable to have a decision with a 95% confidence interval. There would be a high risk associated with someone passing the test who should have failed. In the latter case, the true score is in between 2 times (1.96 actually) the SEM above and below the actual observed score of the examinee. If the lower bound of the confidence interval passes the actual cut-score set or in other words is below the cut-score, the examinee should be considered as a fail. Another way of interpreting the cut-score correctly is by adding the 2*SEM to the cut-score to prevent false positive scores (students who pass that should have been considered as a fail due to error).
What is Smart Data Analysis?
A symposium session at this year’s AMEE conference was titled “Understanding student behaviour: The role of digital data”. This left me wondering about the reliability of the initial data used for big data analysis. We will soon publish research which shows the Standard Error of Measurement of 9 European Universities differs considerably. In this study, the error margins of the 95% Confidence Intervals vary up to 22% below and above the observed score. During this session, many questions were asked about this ‘big data’, for example, which data specifically, how secure is it etc.
In my opinion, we need to move on from big data analysis to SMART Analysis. In my work with Qpercom, we will focus on those scores above the 68% e.g. 95% Confidence Interval (of any exam), in between the 1 and/or 2 SEM, and below the 1 or 2 SEM borders. The predictive value (good marks predict good doctorship) might well be different for each of these outcome levels. An enhancement to our current systems, Qpercom Analyse, will provide this analysis with multiple presentation options to share within teams. Whilst it is not always a prioritised or favoured outcome, students deserve standard setting and quality assurance from their assessors.